The KAI Data Center Builder workload emulation solution replicates the network communication patterns of real-world AI training jobs.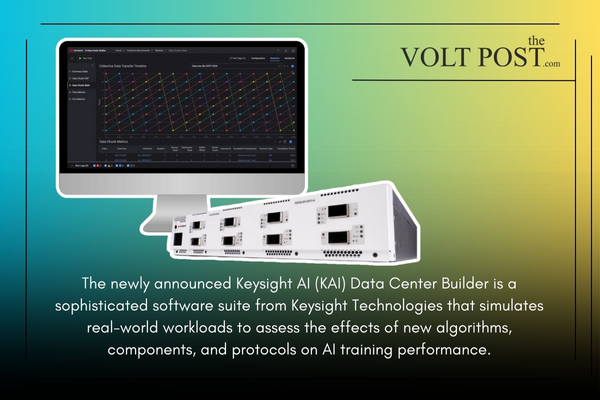
The newly announced Keysight AI (KAI) Data Center Builder is a sophisticated software suite from Keysight Technologies that simulates real-world workloads to assess the effects of new algorithms, components, and protocols on AI training performance.
Large language model (LLM) and other artificial intelligence (AI) model training workloads can be integrated into the design and validation of networks, hosts, and accelerators using KAI Data Center Builder’s workload emulation feature.
System performance is increased by this technique, which makes it possible for hardware design, protocols, architectures, and AI training algorithms to work more closely together.
To speed up AI model training, AI operators employ a variety of parallel processing techniques, commonly referred to as model partitioning. Training performance is improved when model partitioning is in line with the AI cluster structure and setup.
Experimentation is the greatest way to answer important issues during the AI cluster design phase. The effectiveness of data transfer between the graphics processing units (GPUs) is the subject of many of the questions. Important things to think about are:
- Scale-up design of GPU interconnects inside an AI host or rack
- Scale-out network design, including bandwidth per GPU and topology
- Configuration of network load balancing and congestion control
- Tuning of the training framework parameters
In order to speed up experimentation, lower the learning curve required for proficiency, and offer deeper insights into the cause of performance degradation—all of which are difficult to accomplish with real AI training jobs alone—the KAI Data Center Builder workload emulation solution replicates the network communication patterns of real-world AI training jobs.
Customers of Keysight have access to a library of LLM workloads, including GPT and Llama, along with a number of well-known model partitioning schemas, including three-dimensional (3D) parallelism, Data Parallel (DP), and Fully Sharded Data Parallel (FSDP).
Using the workload emulation application in the KAI Data Center Builder enables AI operators to:
- Experiment with parallelism parameters, including partition sizes and their distribution over the available AI infrastructure (scheduling)
- Understand the impact of communications within and among partitions on overall job completion time (JCT)
- Identify low-performing collective operations and drill down to identify bottlenecks
- Analyze network utilization, tail latency, and congestion to understand the impact they have on JCT
With the help of the KAI Data Center Builder’s new workload emulation features, infrastructure vendors, AI operators, and GPU cloud providers can evaluate the developing designs of AI clusters and new components by introducing realistic AI workloads into their lab setups.
In order to optimize the infrastructure and boost the performance of AI workloads, they can also experiment with fine-tuning model partitioning schemas, parameters, and algorithms.
By validating AI cluster components using real-world AI workload emulation, KAI Data Center Builder serves as the cornerstone of the Keysight Artificial Intelligence (KAI) architecture, a portfolio of end-to-end solutions intended to assist clients in scaling artificial intelligence processing capacity in data centers.
Leadership Comments
Ram Periakaruppan, Vice President and General Manager, Network Test & Security Solutions, Keysight, said: “As AI infrastructure grows in scale and complexity, the need for full-stack validation and optimization becomes crucial. To avoid costly delays and rework, it’s essential to shift validation to earlier phases of the design and manufacturing cycle. KAI Data Center Builder’s workload emulation brings a new level of realism to AI component and system design, optimizing workloads for peak performance.”
Keysight will showcase KAI Data Center Builder and its workload emulation capabilities in booth #1301 at the OFC 2025 conference, April 1-3, at the Moscone Center, San Francisco, California.