The rapid growth of AI is impacting large data centers as high volumes of traffic and intensive processing requirements are pushing the limits of hyperscale data centers. To successfully support AI’s rapid growth, data center architectures and the high-speed networks they rely on, must be re-evaluated.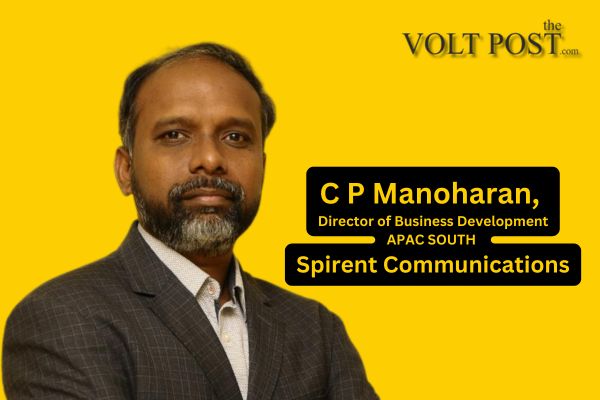
AI application model complexity and size dictate the level of compute, memory and network type needed to connect AI accelerators (like GPUs) used for training and inferencing. At the same time, AI workloads are driving an unprecedented demand for low latency and high bandwidth connectivity between servers, storage, and accelerators.
The scale required for support doesn’t come from simply adding racks to a data center. Handling large AI training and inference workloads requires a separate, scalable, routable backend network infrastructure to connect distributed GPU nodes. AI apps have less impact on the frontend Ethernet networks that use general purpose servers to provide AI data ingestion for the training process.
The requirements for new backend network differ considerably from traditional data center frontend access networks. In addition to higher traffic and increased network bandwidth per accelerator, the backend network needs to support thousands of synchronized parallel jobs, as well as data and compute-intensive workloads.
The network must be scalable, and provide low latency and high bandwidth connectivity between servers, storage, and the GPUs essential for AI training and inferencing.
Data Center Networks Must Transform to Support New AI Workloads
The AI data center journey is just beginning and will change dramatically as AI evolves, promising to be transformative and expensive. Data center architectures should be evaluated sooner rather than later, as new strategies will be required for success of data center networks.
GenAI applications are poised to accelerate a new era of high-speed Ethernet backend for data center networks, as well as other emerging technologies.
Field deployments of 400G Ethernet have started, 800G chipsets are being manufactured, and standards specifications are in development for 1.6 Terabit Ethernet, with each iteration representing a doubling of bandwidth. Backend AI networks are projected to migrate quickly to nearly all port speeds being at 800 Gbps and above by 2027, with triple-digit CAGR for bandwidth growth.
New Ethernet Technologies for Better AI networking
For large-scale AI deployments, latency sensitivities can have a large impact on training performance, so a more deterministic flow control approach can be taken with InfiniBand. High-speed Ethernet and InfiniBand are expected to coexist in data center networks backend for the foreseeable future.
Many organizations have begun deploying 400G and 800G with the RoCE v2 advanced protocol (RDMA over converged Ethernet, version 2) as the data center switch fabric.
This low-cost data transfer network increases efficiency and improves CPU utilization and network performance, while reducing network latency and increasing bandwidth availability.
A Sound Test and Assurance Strategy is the Gateway to AI Success
Test solutions help validate that the industry is leveraging the cost-intensive AI/ML infrastructure to its maximum capabilities and organizations can safely unlock AI’s full potential and transform marginal gains into monumental results by:
Quantifying use cases –
- Identify AI-powered use cases that offer clear business outcomes where quality datasets are available.
- Use digital twins to cost-efficiently and rapidly test use case efficacy and value, and provide feedback loops for continuous AI model learning.
Developing a data architecture and management strategy –
- Data architecture, management, and hygiene should be addressed at an early stage to avoid cost shock, poor data quality, and inaccurate or biased AI models.
- Validate that data center interconnect architectures can cope with the volume of data and high-speed data transfers and access required by AI learning and inference clusters. Consider 400G/800G Ethernet supporting RoCE v2 to address the requirements of high performance, low latency, and a low-cost data transfer network.
- Use real test data to accelerate AI model training with realistic scenarios and unique variations relevant to intended environments.
- Use continuous test data from the live network to keep AI models current.
Pursuing Automation –
- Invest in an automation framework first, integrating AI to enhance and supercharge related processes.
- Start with lower-risk internal processes and environments like labs and test beds to intelligently automate repetitive tasks, streamline complex processes and reduce human errors.
Ensuring Efficacy –
- AI and especially Generative AI (which is in its infancy) can present inaccurate information as though it were correct. While bad or erroneous data can be blamed, so can misalignment with desired business outcomes.
- Continuously verify AI recommendation efficacy against golden scenarios and desired outcomes while providing closed-loop feedback for learning.
- Use digital twins to provide a safe and realistic offline validation environment.
- Use active testing in the operational networks to rapidly verify implemented recommendations and provide feedback loops for reinforcement or to trigger resolutions.
Testing security –
- Explore using modern security solutions that are evolving to utilize AI to enhance their effectiveness and to counter AI-generated attacks.
- Continuously test the efficacy of those security solutions for threat detection, false positives, prevention, and remediation response using hyper-realistic attacks, hacker attack behavior, and evasion techniques.